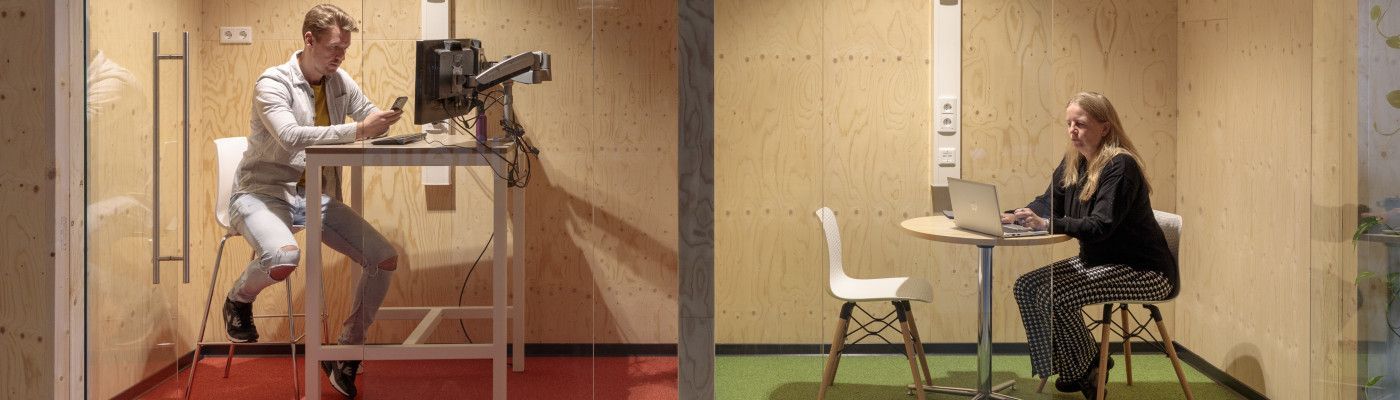
Export
During working hours, export requests are generally processed within a couple of hours
A typical Lifelines dataset contains personal information that is pseudonymized but can still indirectly identify participants. To minimize the risk of identifiable information leaving our research environment (and thereby violating the privacy rights of participants), Lifelines enforces a zero-tolerance policy for raw data or pseudonyms and maintains a standard exportation guideline.
Process
As a researcher working on the Digital Research Environment, you can submit a download request from within the DRE. When working on the UMCG high performance cluster, you can email the Lifelines Data Managers to check whether an export is in order. Please provide the path where the files are located, and the specific file names. The Lifelines Data Management team tries to process your requests within a couple of hours on working days.
Important: make sure your export complies with the export guidelines from Lifelines.
Zero-tolerance on the export of personal data
Please do not attempt to export any personal data (including pseudonyms) from your research environment yourself, for example by making a screenshot or copying them manually (typing or writing). To protect the personal data and privacy of our participants, Lifelines enforces a zero-tolerance policy on such exports, independent of the receiver or the identifiability of the exported personal data. In case of doubt, please contact one of the Lifelines Data Managers.
Guidelines for requested exports
To protect the personal data and privacy of our participants, Lifelines maintains the general rule that the minimal participant group size for which results can be exported or published is N = 10. This rule minimizes the risk that individual participants can be recognized (by themselves or by third parties) based on the reported results, which in turn may lead to unwanted consequences, such as misuse of sensitive information for commercial or political reasons, or participants involuntary learning about personal health-related information. See below for examples and advice on how to adhere to our export rule.
Three important additions:
- If you use the Lifelines UMCG HPC as your research environment, you are technically able to export files yourself. However, you are contractually (D(M)TA + CoC) bound to follow the two criteria above. In case you are in doubt whether you can export a file, contact one of the Lifelines Data Managers.
- In case of a request for a transfer between two Lifelines projects, please look at the specifications on the Import page.
- In some cases it might be difficult to adhere to the standard export guideline. In these instances we will consider a possible exception for your request. Please include at least the points listed below when submitting your request (for information see document below). Note: a download request from the DRE leaves room for only a small justification. In the justification field, please refer to your email and send an email to the Lifelines Data Managers providing the necessary information.
- That your export request does not meet the standard export guideline N>= 10. Please specify all tables/figures/sections in your export/manuscript for which this is the case (but keep our zero tolerance rule in mind).
- Why you are unable to remove each small group size.
- Description of all selection criteria, i.e., characteristics that participants need to have in order to be part of the group.
- The result (finding) itself: is this something Lifelines participants are likely to know about themselves or not?
Examples of adhering to the standard export guideline
Figures
You can request the export of figures from your research environment. However, please consider how identifying the values might be. Especially the depiction of outliers might risk the identification of our participants (see example figure). The example figure shows a scatterplot, however, the same applies for other figures like boxplots, histograms, or bar charts. The only solution to such an issue is to remove the outliers or the complete figure.

Tables
In the table shown on the right, several of the presented results describe participant groups that are smaller than N = 10 (data are fictive). To adhere to our guidelines, we will ask you to alter your table. Below we present several possible adjustment methods.

Solution 1: Aggregating your results
One of the possible solutions is to combine two (or more) small groups into one group. In our example this would entail combining age groups: combining the age groups <20 and 20-30 together, and the same for the age groups 41-50 and 51+.

Solution 2: replacing small results with <10
A second possible solution is to replace the small observations with "<10". This way, the reader and participants do not know the exact amount of participants presented. Make sure to also replace the zero values with <10. Important note: please make sure that the other group sizes mentioned in the table cannot be used to calculate the obscured group size!
In our example, this solution works well for the ex-smoker category, as there are several age groups with N<10. However, we are able to calculate the exact number for the current smoker category by substracting all other age groups from the total number in the header. There are two ways to resolve the issue with the current smoker category:
- Option 1: removing the total numbers by the smoking categories. As a reader you shouldn't be able to find the number somewhere else in the table either.
- Option 2: add uncertainty to an additional age group (in this example age group 20-30).


Solution 3: presenting percentages instead of absolute values
The third solution is to present results as percentages from the total group in your table, instead of exact group sizes. Important note: please carefully check on the possibility to calculate the exact group size.
In our example, the exact numbers are still traceable as the total size of the smoker groups are relatively small. There are two ways to resolve this issue:
- Option 1: remove the total group sizes.
- Option 2: display the results as "smaller than" the percentage for a group size of N = 10.


Solution 4: leaving out a category
The final solution is to leave out categories that or not of interest to you. By doing so, the observations with "<10" are not traceable from the total minus the frequencies in the other categories.
In our example, you might be interested in younger and older participants only. As a result you could leave out the age groups 20-30 and 31-40. The same principle would apply when your categories are yes and no (leave out the no or yes so that the exact number cannot be deduced).
